How does the brain’s function emerge from its underlying structure?
The relationship between the brain’s anatomy – how bundles of neurons connect disparate regions in complex patterns – to the brain’s function – how those disparate regions coordinate with each other across time to produce cognition, behavior, and states of disease – is of fundamental importance in neuroscience.
It turns out the brain’s structure is only slightly correlated to its function, meaning that the relationship is not simply one-to-one, but is instead governed by more subtle and complex mechanisms. The key feature that we use to model this complex emergence is a network diffusion model, which describes how signals travel through the brain’s structure (Abdelnour et al., 2014).
Overview of brain structure-function modeling
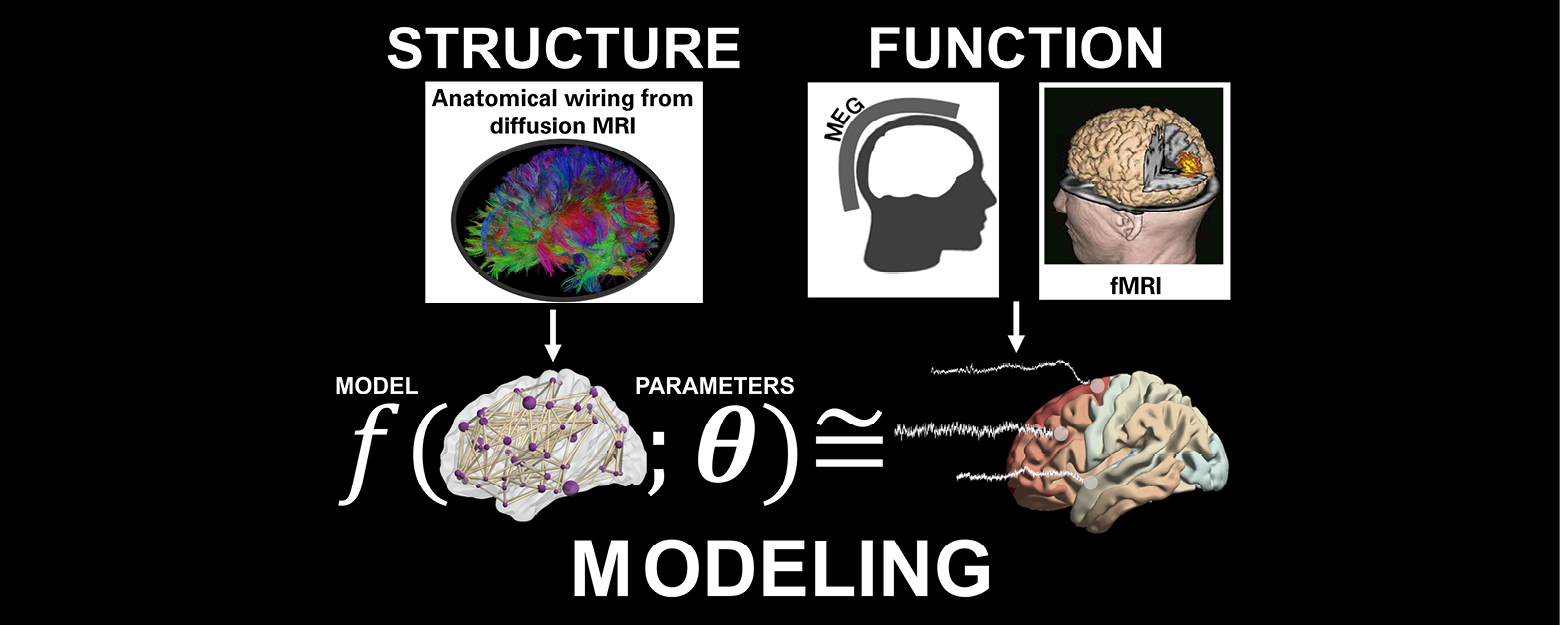
This framework assumes that signals don’t know where they are in the brain, and instead they diffuse along paths of least resistance (aka “random-walks”) throughout the brain. In further work, we’ve shown that the brain’s eigenmodes (also known as “harmonics,” which are fundamental patterns that describe a network) are deeply related to and can help predict the brain’s function (Abdenlour et al., 2018). We find that these network diffusion models (represented by closed-form equations) work surprisingly well to describe functional networks emerging from the brain's structure, but a significant challenge is to relate aspects of these models to the underlying biology.
Thus, a key advance in some of our lab’s latest work is to build Spectral Graph Models (“SGM”) (Raj et al., 2020; Verma et al., 2022, Verma et al., 2022) that can predict the brain’s function through biophysically interpretable parameters, such as with neural time-constants and neural excitatory/inhibitory gains. These currently work well with magnetoencephalography (MEG) data, which can capture the brain’s rich frequency spectra. Through this model, we have found significant differences in the model’s parameters that relate healthy brains to Alzheimer’s disease (Ranasinghe et al., 2022, Verma et al., Preprint). However, an active area of our present research is to extend SGM to fMRI functional connectivity (Raj et al., in submission) and to functional connectivity defined by MEG.
Our current outlook involves continuing to innovate and improve the SGM model, finding relationships between SGM parameters and disease states, unveiling model parameters’ relationship to dynamic functional connectivity, and expanding the model to incorporate information about the brain’s distribution of cell-type.
Basics of the Structure-Function Relationship
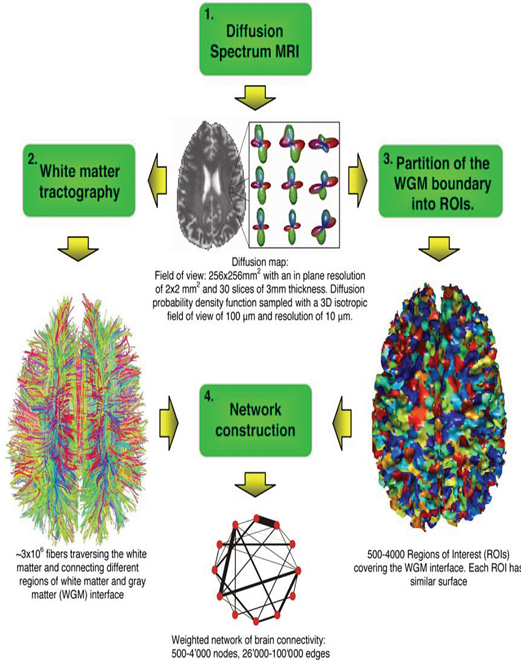
The brain is a highly complex system, and it is akin to a network, structurally and functionally.
The former can be compared to a city’s map, indicating the streets from neighborhood A to neighborhood B. The map may furthermore indicate the width or number of lanes of each street.
Likewise, the brain’s white matter provides connections between the different parts of the brain. At a crude scale of 90 or 86 regions, the dense connections are modeled as a matrix indicating the connection’s strength between any two regions (or “neighborhoods”).
Similarly, the town’s neighborhoods may depend on each other in some functional manner, perhaps one neighborhood is known for its restaurants, frequented by workers from a neighborhood known for its financial companies. This is a functional relationship between the two neighborhoods. One can then construct a functional connectivity between the town’s neighborhoods.
Likewise, we construct functional networks of the brain obtained from the measured activities of the various regions of the brain.
In computational neuroscience we seek a relationship between function and structure such that one can be determined from the other.