Predicting Neurodegenerative Disease with Network Models
Neurodegenerative diseases (NDDs), including Alzheimer’s disease (AD), Parkinson’s disease (PD), frontotemporal lobar dementia (FTLD), Huntington’s disease (HD), and amyotrophic lateral sclerosis (ALS), are all similar in that they are progressive, resistant to treatment, and universally fatal. While we have elucidated many key facets of the pathophysiology of these diseases over recent decades, there is much we still do not understand. A multidisciplinary approach that includes clinical research, bench science, and computational modeling will be required to open new avenues for improving the lives of the millions of people suffering from NDDs worldwide.
Computational Modeling
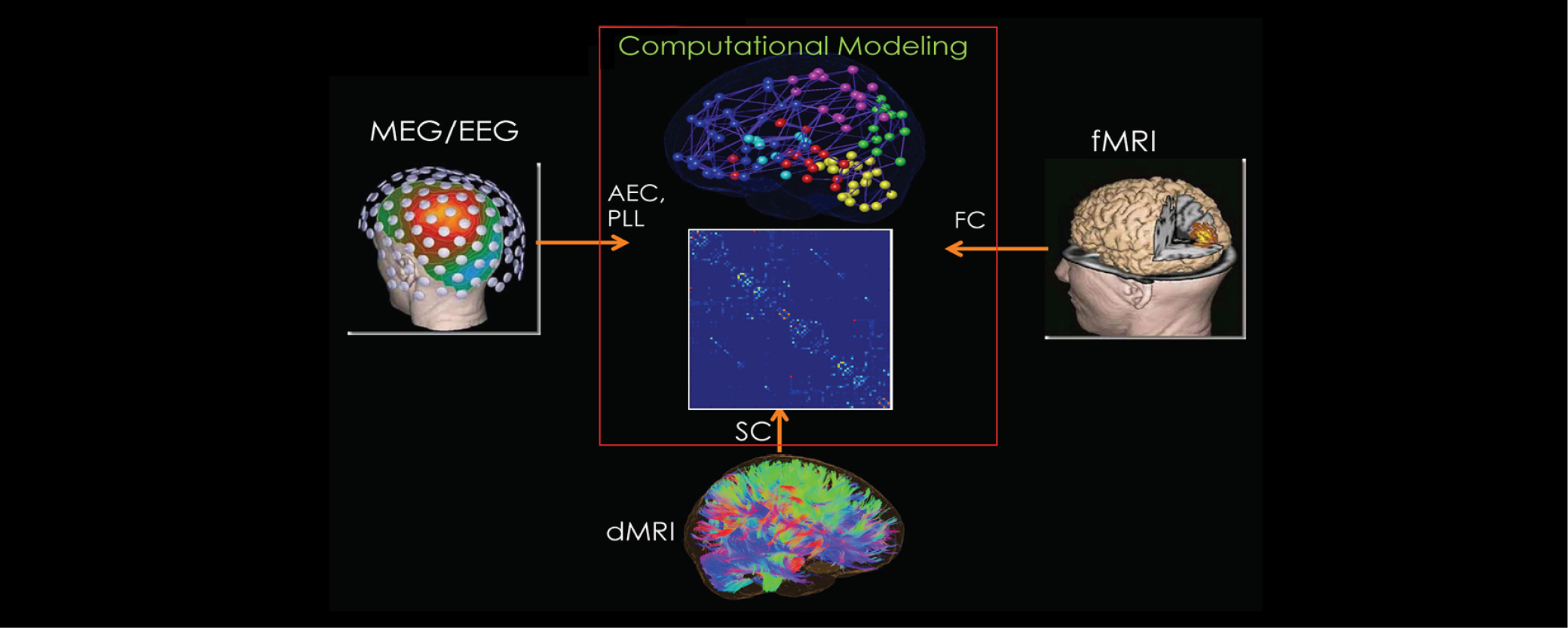
One important pathophysiological feature common to most, if not all, NDDs is that they are prion-like; that is, the key mediators of pathological changes in the brain are agglomerates of misfolded proteins that accumulate and spread trans-neuronally. For example, the brains of AD patients harbor two kinds of ‘proteinopathies’: amyloid-β (Aβ), which forms extracellular plaques, and tau (τ), which forms intracellular neurofibrillary tangles (NFTs). Similarly, the main protein implicated in PD is ɑ-synuclein (ɑ-syn). These pathological protein species are thought to first form in ‘canonical’ initiation sites (e.g., the entorhinal cortex in AD and the substantia nigra in PD) and then spread along the brain’s white matter tracts to structurally connected regions. In other words, the whole-brain connectome can be thought of as a network of highways along which NDD pathology spreads. Therefore, it should be possible to describe the progression of NDDs using graph theory and biophysical models.
Our lab was the first to develop a mathematical model of this network-based spread of proteinopathy. This model, called the Network Diffusion Model (NDM), is a first-order, homogeneous set of differential equations that poses the following: the rate of spread of a protein species between each pair of brain regions is proportional to the protein’s concentration difference as well as the amount of connectivity the pair shares. It has been used to accurately model the longitudinal progression of pathology in AD, PD, and ALS as well as mouse models of NDDs. We have further used this model to go back in time and infer the likeliest initiation sites of AD and PD.
While our lab and others have employed NDM-type models to great effect, they are incomplete descriptions of the disease process. Our lab has recently begun to develop and employ more sophisticated mathematical models that are capable of explaining more facets of NDD pathophysiology. These models have two major aims: 1) provide a platform for identifying or validating key factors important to the development of brain pathology (e.g., AD risk gene expression) in the context of network spread, and 2) predict the progression of brain pathology with more accuracy than existing methods. Below we detail ongoing approaches to accomplish these goals.
Featured Publications
Network Models of Neurological Diseases
- Regional transcriptional architecture of Parkinson's disease pathogenesis and network spread
https://pubmed.ncbi.nlm.nih.gov/31359041/ - A method for inferring regional origins of neurodegeneration
https://pubmed.ncbi.nlm.nih.gov/29409009/ - Network diffusion model of progression predicts longitudinal patterns of atrophy and metabolism in Alzheimer’s Disease.
https://pubmed.ncbi.nlm.nih.gov/25600871/ - A Network Diffusion model of disease progression in dementia
https://pubmed.ncbi.nlm.nih.gov/22445347/
Network Models of Brain Activity and “Graph Harmonics”
- A joint subspace mapping between structural and functional brain connectomes.
https://pubmed.ncbi.nlm.nih.gov/36870432/ - Time-varying dynamic network model for dynamic resting state functional connectivity in fMRI and MEG imaging.
https://pubmed.ncbi.nlm.nih.gov/35337963/ - Spectral graph theory of brain oscillations--Revisited and improved
https://pubmed.ncbi.nlm.nih.gov/35051584/ - Spectral graph theory of brain oscillations.
https://pubmed.ncbi.nlm.nih.gov/35051584/ - Brain Network Eigenmodes Provide a Robust and Compact Representation of the Structural Connectome in Health and Disease.
https://pubmed.ncbi.nlm.nih.gov/28640803/
Brain Spatial Transcriptomics
- Matrix Inversion and Subset Selection (MISS): Pipeline for mapping of diverse cell types across the murine brain.
https://pubmed.ncbi.nlm.nih.gov/35363567/ - Modeling seeding and neuroanatomic spread of pathology in Amyotrophic Lateral Sclerosis.
https://pubmed.ncbi.nlm.nih.gov/35143975/ - Origins of atrophy in Parkinson linked to early onset and local transcription patterns.
https://pubmed.ncbi.nlm.nih.gov/32954322/ - Regional vulnerability in Alzheimer’s: The role of cell-autonomous and trans-neuronal processes.
https://alz-journals.onlinelibrary.wiley.com/doi/10.1016/j.jalz.2017.11.014