Researchers at the Raj Lab are applying machine learning approaches to multiple research fields, including Alzheimer’s Disease pathology, dementia progression, cell type analysis, and more.
The key to successful machine learning studies is the strong connection between the models’ predictive power and the guidance of domain knowledge. We have experimented with various machine learning and deep learning models, such as Gradient Boosting Trees, Convolutional Neural Networks, UNet, and LSTM.
The main artificial intelligence research project is led by Daren Ma. He developed a novel multi-task UNet model for ADNI subjects’ diagnosis and cognition prediction using their baseline sMRI brain scans. This work was then expanded to other meaningful reaches such as longitudinal prediction, brain parcellation, and age prediction. Now the team is working hard to build a healthy control standard group for the brain age model, collecting data from UK Biobank plus HABS databases.
UNet Model
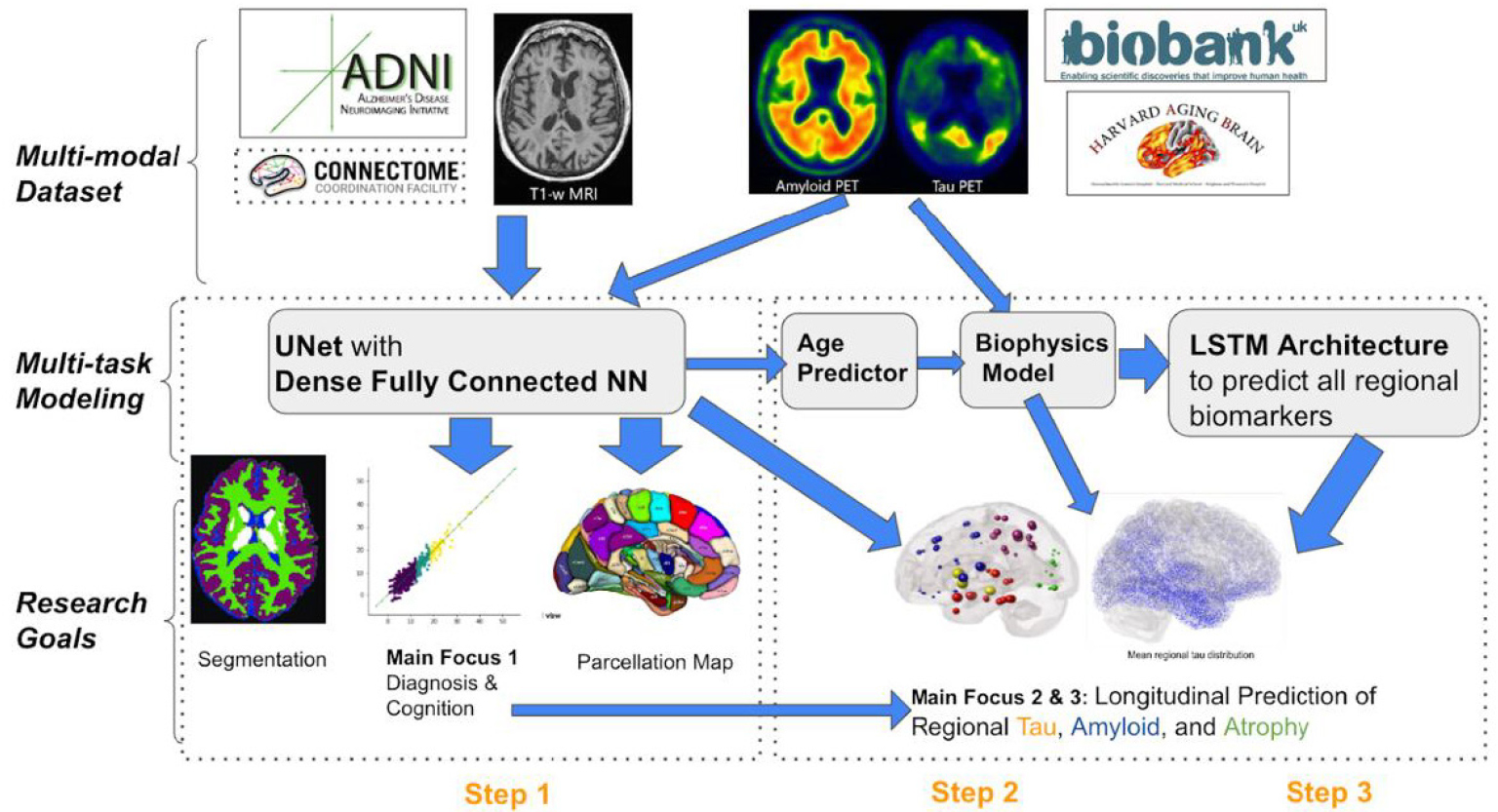