As experimental tools become ever more refined, we are fast approaching the realization of a key goal in basic neuroscience: determining how the brain is organized at the single-cell level. Such a “cytoarchitectonic atlas,” which provides not only the locations of cell bodies and their projections, but also their cell-type identities, would represent a quantum leap in our ability to discern the structural and functional organization of the brain.
While single-cell atlases of fruit flies and zebrafish have been developed, mammalian brains remain too large and complex to fully map at this resolution. Excitingly, there have been recent developments in spatial transcriptomics that have provided cell-type maps of several regions of the mouse brain. However, while there is much to be gleaned from the data currently available, these techniques have not yet been scaled up to produce a whole-brain cytoarchitectonic atlas in a mammalian brain.
To fill this gap in knowledge, our lab has recently developed and published a computational method for developing a whole-brain cell-type atlas in the mouse: Matrix Inversion and Subset Selection (MISS). We leverage publicly available single-cell RNA sequencing (scRNAseq) data, sampled from discrete brain regions, and the Allen Gene Expression Atlas (AGEA), which mapped the expression of thousands of genes at an isotropic resolution of 200 μm using in situ hybridization (ISH).
Whole-Brain Cell-Type Atlas
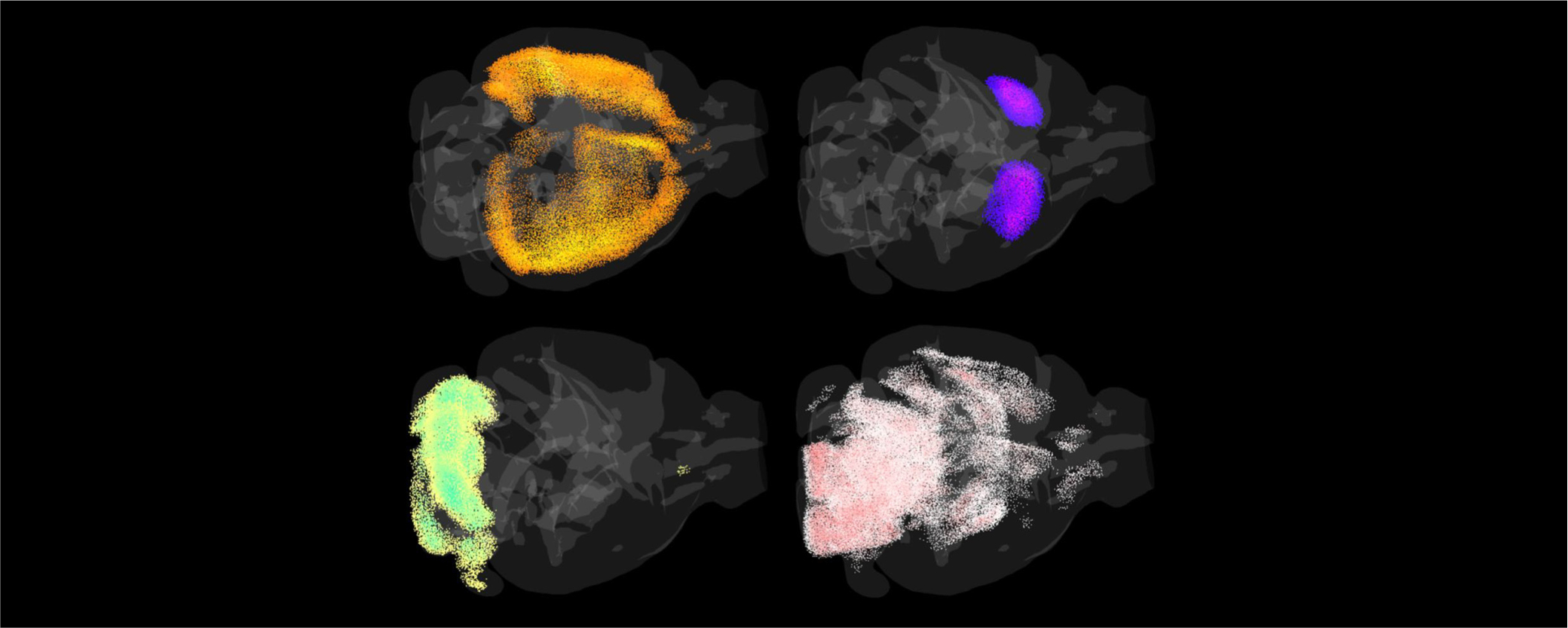
The algorithm takes place in two steps:
- We use a filtering algorithm called MRx3 to determine a maximally informative subset of genes, tailored to the cell types contained in the scRNAseq dataset.
- We use this gene subset to perform a spatial deconvolution of the AGEA, under the assumption that the total gene expression in each 200-μm voxel can be represented by a linear combination of the gene expression profiles of cell types. We use nonnegative matrix inversion to determine these densities voxel-by-voxel. We have demonstrated that MISS is both accurate and robust in mapping a wide variety of neuronal and non-neuronal cell types using three separate scRNAseq datasets.
Our current cell-type mapping work has two major aims:
- We are developing more refined methods for performing both the subset selection and matrix inversion halves of MISS, which we dub “MISS 2.0.”
- We are attempting to apply MISS on the scRNAseq and spatial transcriptomic data available in humans.
While the field is rapidly converging on a whole-brain mouse cytoarchitectonic atlas, we anticipate that resolving a cell-type atlas of the human brain, whose billions of individual cells are far too numerous to fully map even with current state-of-the-art techniques, will have tremendous value both for basic neuroscience and clinical research.
Modeling Selective Cell-Type Vulnerability
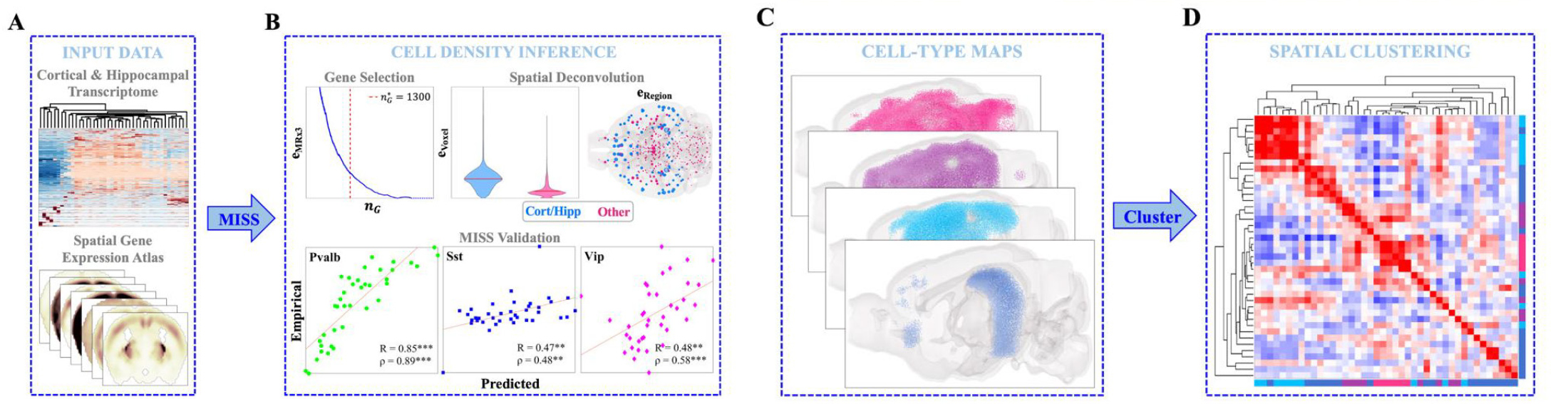
Cell-Type Density Inference with Spatial Deconvolution
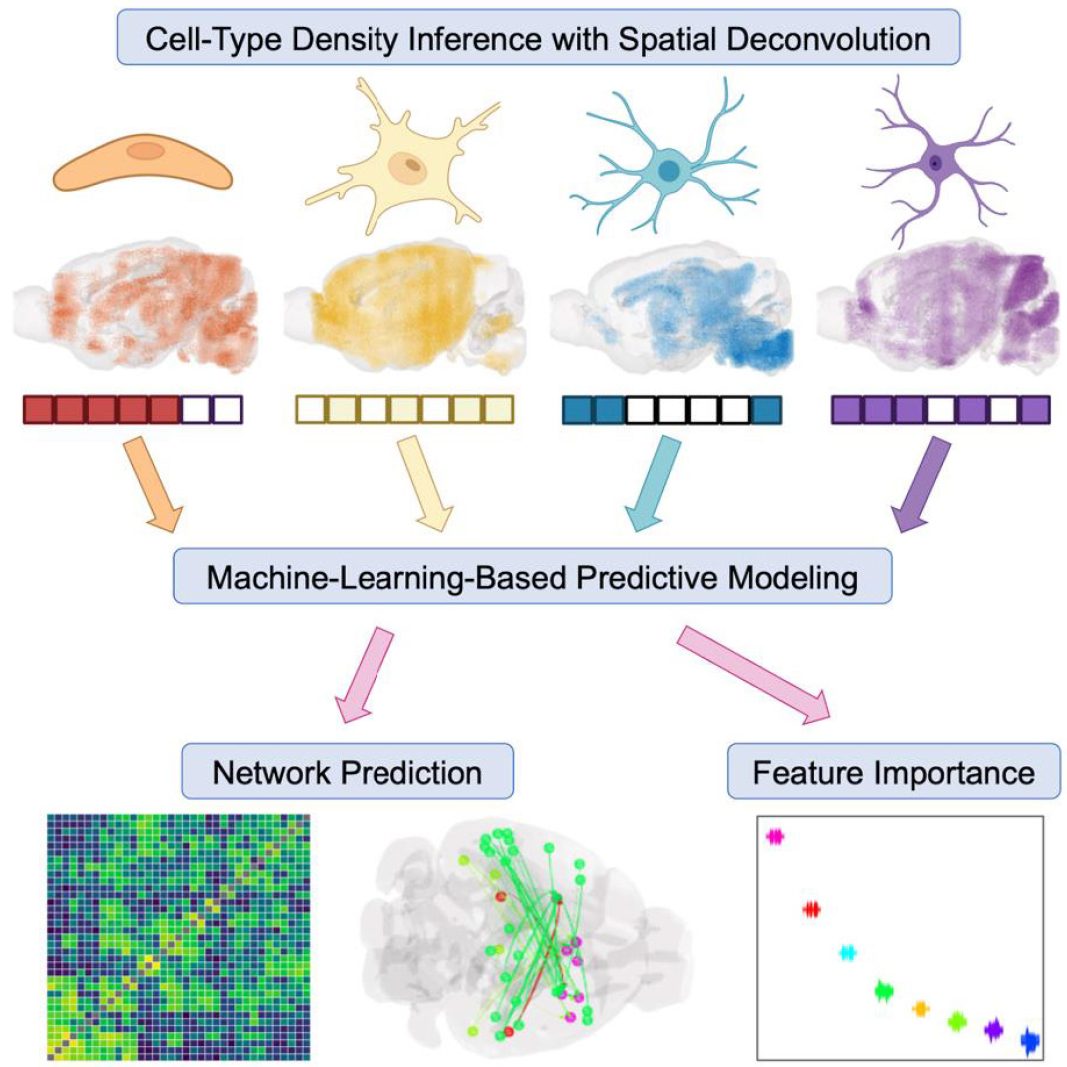